Speaker: Andreas Ekström.
Organized by: CHAIR theme AI for Scientific Data Analysis.
Overview
- Date:Starts 8 June 2023, 13:00Ends 8 June 2023, 15:00
- Location:
- Language:English
Data-driven emulators based on Gaussian processes and neural networks represent common methods in machine learning for developing fast and accurate surrogate models that mimic the output of complex computer simulations.
In this seminar I will focus on the design and development of model-driven emulators that exploit the structure of the underlying equations of the simulator. This type of emulators offer tremendous accuracy and efficiency.
Eigenvector continuation is one such method that has been developed by the low-energy nuclear theory community over the past few years. It turns out that we "reinvented a wheel” and eigenvector continuation already exists as a subset of reduced-basis methods dating back to the late 1970s. Nevertheless, this class of emulators is a game-changer that opens up very exciting Bayesian analyses of quantum many-body systems like atomic nuclei.
Speaker
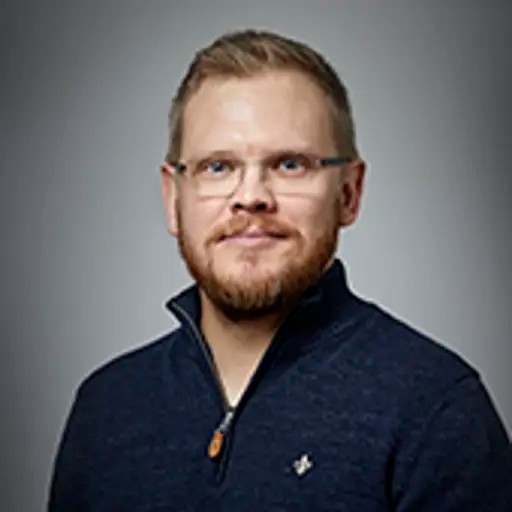
- Professor, Subatomic, High Energy and Plasma Physics, Physics
CHAIR contact

- Doctoral Student, Institution of physics at Gothenburg University
CHAIR theme

AI for Scientific Data Analysis
This theme is about utilizing the power of AI as a tool for scientific research. AI can be applied to, and potentially speed up, discovery and utilization in a variety of research disciplines, such as microscopy, physics, biology, chemistry, and astronomy.