Interpretable AI is an emerging field, focused on developing AI systems that are transparent and understandable to humans.

Many currently popular AI systems operate as black boxes, meaning that it is hard to understand how they make their decisions and to correct errors that inevitably occur from time to time. In many situations, black box AI systems have also been shown to maintain or even enhance biases present in their training data. By contrast, interpretable AI models are designed in a transparent manner, making it possible for a human observer to follow (and, if needed, correct) their decision-making processes.
This is especially important in applications where decisions involve high stakes or legal implications, such as healthcare, personal finance, or traffic applications. Interpretable AI models can also help increase trust in AI systems, making it easier for humans to work alongside them.
The CHAIR theme Interpretable AI executes a series of activities, including seminars, workshops, and research projects, that aim to develop, extend, study, and compare interpretable methods and to contrast them with black box models. The projects cover a range of applications, such as conversational AI, forestry management, and multi-vehicle trajectory planning, and involve collaborations with researchers from various fields.
Events 2023
- A bird's eye view on Responsible AI
- Interpretable approaches in human-machine interaction
- Case-based reasoning in AI: interpretability, explanations, and sharing best practices
- Toward Safe and Efficient Human-Robot Teams: Understanding Robot Motion and Safety using Mixed Reality
- Two key challenges for robot learning in human-robot interaction: Understanding social contexts and scaling human supervision
Events 2024
- Learning interpretable-by-design models within latent deep foundation feature spaces
- Safety, Adaptation and Efficient Learning in Physical Human-Robot Interaction: A Dynamical Systems Approach
- Learning for Adaptive and Reactive Robot Control
- Toward Concept-Based Explanations for Intelligent Systems
- Interpretable Artificial Intelligence Through Glass-Box Models (Opens in new tab)
Theme leader
Leadership team

- Senior Lecturer, Vehicle Engineering and Autonomous Systems, Mechanics and Maritime Sciences
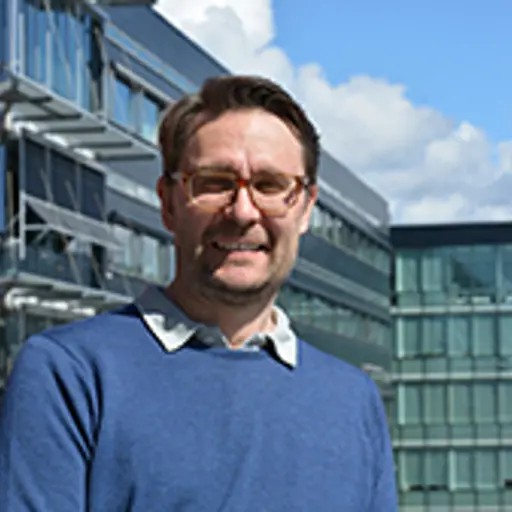
- Full Professor, Interaction Design and Software Engineering, Computer Science and Engineering

- Associate Professor, Computer and Network Systems, Computer Science and Engineering
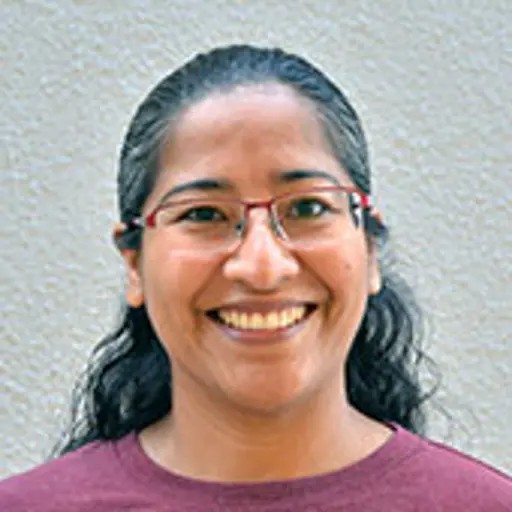
- Associate Professor, Systems and Control, Electrical Engineering

- Head of Division, Data Science and AI, Computer Science and Engineering